GenomicRanges
: Genomic analysis¶
GenomicRanges
is a Python package designed to handle genomic locations and facilitate genomic analysis. It is similar to Bioconductor’s GenomicRanges and uses the IRanges package under the hood to manage and provide interval-based arithmetic operations.
An IRanges
holds a start position and a width, and is typically used to represent coordinates along a genomic sequence. The interpretation of the start position depends on the application; for sequences, the start is usually a 1-based position, but other use cases may allow zero or even negative values, e.g., circular genomes. Ends are considered inclusive. IRanges
uses nested containment lists under the hood to perform fast overlap and search-based operations.
The package provides a GenomicRanges
class to specify multiple genomic elements, typically where genes start and end. Genes are themselves made of many subregions, such as exons, and a GenomicRangesList
enables the representation of this nested structure.
Moreover, the package also provides a SeqInfo
class to update or modify sequence information stored in the object. Learn more about this in the GenomeInfoDb package.
The GenomicRanges
class is designed to seamlessly operate with upstream packages like RangeSummarizedExperiment
or SingleCellExperiment
representations, providing consistent and stable functionality.
Note
These classes follow a functional paradigm for accessing or setting properties, with further details discussed in functional paradigm section.
Installation¶
To get started, install the package from PyPI
pip install genomicranges
Construct a GenomicRanges
object¶
We support multiple ways to initialize a GenomicRanges
object.
From Bioinformatic file formats¶
From biobear
¶
Although the parsing capabilities in this package are limited, the biobear library is designed for reading and searching various bioinformatics file formats, including FASTA, FASTQ, VCF, BAM, and GFF, or from an object store like S3. Users can esily convert these representations to GenomicRanges
(or read more here):
from genomicranges import GenomicRanges
import biobear as bb
session = bb.new_session()
df = session.read_gtf_file("path/to/test.gtf").to_polars()
df = df.rename({"seqname": "seqnames", "start": "starts", "end": "ends"})
gg = GenomicRanges.from_polars(df)
# do stuff w/ a genomic ranges
print(len(gg), len(df))
From UCSC or GTF file¶
You can also import genomes from UCSC or load a genome annotation from a GTF file. This requires installation of additional packages pandas and joblib to parse and extract various attributes from the gtf file.
import genomicranges
# gr = genomicranges.read_gtf(<PATH TO GTF>)
# OR
human_gr = genomicranges.read_ucsc(genome="hg19")
print(human_gr)
Preferred way¶
To construct a GenomicRanges
object, we need to provide sequence information and genomic coordinates. This is achieved through the combination of the seqnames
and ranges
parameters. Additionally, you have the option to specify the strand
, represented as a list of “+” (or 1) for the forward strand, “-” (or -1) for the reverse strand, or “*” (or 0) if the strand is unknown. You can also provide a NumPy vector that utilizes either the string or numeric representation to specify the strand
. Optionally, you can use the mcols
parameter to provide additional metadata about each genomic region.
from genomicranges import GenomicRanges
from iranges import IRanges
from biocframe import BiocFrame
from random import random
gr = GenomicRanges(
seqnames=[
"chr1",
"chr2",
"chr3",
"chr2",
"chr3",
],
ranges=IRanges([x for x in range(101, 106)], [11, 21, 25, 30, 5]),
strand=["*", "-", "*", "+", "-"],
mcols=BiocFrame(
{
"score": range(0, 5),
"GC": [random() for _ in range(5)],
}
),
)
print(gr)
GenomicRanges with 5 ranges and 2 metadata columns
seqnames ranges strand score GC
<str> <IRanges> <ndarray[int8]> <range> <list>
[0] chr1 101 - 111 * | 0 0.3770834720856471
[1] chr2 102 - 122 - | 1 0.8921722889015103
[2] chr3 103 - 127 * | 2 0.3961228666512363
[3] chr2 104 - 133 + | 3 0.9005988816274386
[4] chr3 105 - 109 - | 4 0.15984468514506478
------
seqinfo(3 sequences): chr1 chr2 chr3
Note
The input for mcols
is expected to be a BiocFrame
object and will be converted to a BiocFrame
in case a pandas DataFrame
is supplied.
Pandas DataFrame
¶
If your genomic coordinates are represented as a pandas DataFrame
, convert this into GenomicRanges
if it contains the necessary columns.
Important
The DataFrame
must contain columns seqnames
, starts
and ends
to represent genomic coordinates. The rest of the columns are considered metadata and will be available in the mcols
slot of the GenomicRanges
object.
from genomicranges import GenomicRanges
import pandas as pd
df = pd.DataFrame(
{
"seqnames": ["chr1", "chr2", "chr1", "chr3", "chr2"],
"starts": [101, 102, 103, 104, 109],
"ends": [112, 103, 128, 134, 111],
"strand": ["*", "-", "*", "+", "-"],
"score": range(0, 5),
"GC": [random() for _ in range(5)],
}
)
gr_from_df = GenomicRanges.from_pandas(df)
print(gr_from_df)
GenomicRanges with 5 ranges and 2 metadata columns
seqnames ranges strand score GC
<str> <IRanges> <ndarray[int8]> <list> <list>
0 chr1 101 - 112 * | 0 0.3671818607745383
1 chr2 102 - 103 - | 1 0.4447271765216526
2 chr1 103 - 128 * | 2 0.4094592099350556
3 chr3 104 - 134 + | 3 0.32487574630629934
4 chr2 109 - 111 - | 4 0.6110315121747223
------
seqinfo(3 sequences): chr1 chr2 chr3
Polars DataFrame
¶
Similarly, To initialize from a polars DataFrame
:
from genomicranges import GenomicRanges
import polars as pl
from random import random
df = pl.DataFrame(
{
"seqnames": ["chr1", "chr2", "chr1", "chr3", "chr2"],
"starts": [101, 102, 103, 104, 109],
"ends": [112, 103, 128, 134, 111],
"strand": ["*", "-", "*", "+", "-"],
"score": range(0, 5),
"GC": [random() for _ in range(5)],
}
)
gr = GenomicRanges.from_polars(df)
print(gr)
GenomicRanges with 5 ranges and 2 metadata columns
seqnames ranges strand score GC
<str> <IRanges> <ndarray[int8]> <list> <list>
[0] chr1 101 - 112 * | 0 0.34214583105695073
[1] chr2 102 - 103 - | 1 0.8004113433339617
[2] chr1 103 - 128 * | 2 0.2708948741466033
[3] chr3 104 - 134 + | 3 0.9070203500925637
[4] chr2 109 - 111 - | 4 0.168001796345861
------
seqinfo(3 sequences): chr1 chr2 chr3
Sequence information¶
The package also provides a SeqInfo
class to update or modify sequence information stored in the object. Learn more about this in the GenomeInfoDb package.
from genomicranges import SeqInfo
seq = SeqInfo(
seqnames = ["chr1", "chr2", "chr3"],
seqlengths = [110, 112, 118],
is_circular = [True, True, False],
genome = "hg19",
)
gr_with_seq = gr.set_seqinfo(seq)
print(gr_with_seq)
GenomicRanges with 5 ranges and 2 metadata columns
seqnames ranges strand score GC
<str> <IRanges> <ndarray[int8]> <list> <list>
[0] chr1 101 - 112 * | 0 0.34214583105695073
[1] chr2 102 - 103 - | 1 0.8004113433339617
[2] chr1 103 - 128 * | 2 0.2708948741466033
[3] chr3 104 - 134 + | 3 0.9070203500925637
[4] chr2 109 - 111 - | 4 0.168001796345861
------
seqinfo(3 sequences): chr1 chr2 chr3
Getters/Setters¶
Getters are available to access various attributes using either the property notation or functional style.
# access sequence names
print("seqnames (as property): ", gr.seqnames)
print("seqnames (functional style): ", gr.get_seqnames())
# access all start positions
print("start positions: ", gr.start)
# access annotation information if available
gr.seqinfo
# compute and return the widths of each region
print("width of each region: ", gr.get_width())
# or gr.width
# access mcols
print(gr.mcols)
seqnames (as property): ['chr1', 'chr2', 'chr1', 'chr3', 'chr2']
seqnames (functional style): ['chr1', 'chr2', 'chr1', 'chr3', 'chr2']
start positions: [101 102 103 104 109]
width of each region: [12 2 26 31 3]
BiocFrame with 5 rows and 2 columns
score GC
<list> <list>
[0] 0 0.34214583105695073
[1] 1 0.8004113433339617
[2] 2 0.2708948741466033
[3] 3 0.9070203500925637
[4] 4 0.168001796345861
Setters¶
Important
All property-based setters are in_place
operations, with further details discussed in functional paradigm section.
modified_mcols = gr.mcols.set_column("score", range(1,6))
modified_gr = gr.set_mcols(modified_mcols)
print(modified_gr)
GenomicRanges with 5 ranges and 2 metadata columns
seqnames ranges strand score GC
<str> <IRanges> <ndarray[int8]> <range> <list>
[0] chr1 101 - 112 * | 1 0.34214583105695073
[1] chr2 102 - 103 - | 2 0.8004113433339617
[2] chr1 103 - 128 * | 3 0.2708948741466033
[3] chr3 104 - 134 + | 4 0.9070203500925637
[4] chr2 109 - 111 - | 5 0.168001796345861
------
seqinfo(3 sequences): chr1 chr2 chr3
or use an in-place operation:
gr.mcols.set_column("score", range(1,6), in_place=True)
print(gr.mcols)
BiocFrame with 5 rows and 2 columns
score GC
<range> <list>
[0] 1 0.34214583105695073
[1] 2 0.8004113433339617
[2] 3 0.2708948741466033
[3] 4 0.9070203500925637
[4] 5 0.168001796345861
Access ranges¶
get_ranges()
is a generic method to access only the genomic coordinates:
# or gr.get_ranges()
print(gr.ranges)
IRanges object with 5 ranges and 0 metadata columns
start end width
<ndarray[int32]> <ndarray[int32]> <ndarray[int32]>
[0] 101 112 12
[1] 102 103 2
[2] 103 128 26
[3] 104 134 31
[4] 109 111 3
Subset operations¶
You can subset a GenomicRange
object using the subset ([]
) operator. This operation accepts different slice input types, such as a boolean vector, a slice
object, a list of indices, or names (if available) to subset.
# get the first 3 regions
gr[:3]
# get 1, 3 and 2nd rows
# note: the order is retained in the result
print(gr[[1,3,2]])
GenomicRanges with 3 ranges and 2 metadata columns
seqnames ranges strand score GC
<str> <IRanges> <ndarray[int8]> <list> <list>
[0] chr2 102 - 103 - | 2 0.8004113433339617
[1] chr3 104 - 134 + | 4 0.9070203500925637
[2] chr1 103 - 128 * | 3 0.2708948741466033
------
seqinfo(3 sequences): chr1 chr2 chr3
Iterate over ranges¶
You can iterate over the regions of a GenomicRanges
object. name
is None
if the object does not contain any names. To iterate over the first two ranges:
for name, row in gr[:2]:
print(name, row)
None GenomicRanges with 1 range and 2 metadata columns
seqnames ranges strand score GC
<str> <IRanges> <ndarray[int8]> <list> <list>
[0] chr1 101 - 112 * | 1 0.34214583105695073
------
seqinfo(3 sequences): chr1 chr2 chr3
None GenomicRanges with 1 range and 2 metadata columns
seqnames ranges strand score GC
<str> <IRanges> <ndarray[int8]> <list> <list>
[0] chr2 102 - 103 - | 2 0.8004113433339617
------
seqinfo(3 sequences): chr1 chr2 chr3
Intra-range transformations¶
For detailed description of these methods, refer to either the Bioconductor’s or BiocPy’s documentation.
flank: Flank the intervals based on start or end or both.
shift: Shifts all the ranges specified by the shift argument.
resize: Resizes the ranges to the specified width where either the start, end, or center is used as an anchor.
narrow: Narrows the ranges.
promoters: Promoters generates promoter ranges for each range relative to the TSS. The promoter range is expanded around the TSS according to the upstream and downstream parameters.
restrict: Restricts the ranges to the interval(s) specified by the start and end arguments.
trim: Trims out-of-bound ranges located on non-circular sequences whose length is not
NA
.
gr = GenomicRanges(
seqnames=[
"chr1",
"chr2",
"chr3",
"chr2",
"chr3",
],
ranges=IRanges([x for x in range(101, 106)], [11, 21, 25, 30, 5]),
strand=["*", "-", "*", "+", "-"],
mcols=BiocFrame(
{
"score": range(0, 5),
"GC": [random() for _ in range(5)],
}
),
)
# flank
flanked_gr = gr.flank(width=10, start=False, both=True)
# shift
shifted_gr = gr.shift(shift=10)
# resize
resized_gr = gr.resize(width=10, fix="end", ignore_strand=True)
# narrow
narrow_gr = gr.narrow(end=1, width=1)
# promoters
prom_gr = gr.promoters()
# restrict
restrict_gr = gr.restrict(start=114, end=140, keep_all_ranges=True)
# trim
trimmed_gr = gr.trim()
print("GenomicRanges after the trim operation:")
print(trimmed_gr)
GenomicRanges after the trim operation:
GenomicRanges with 5 ranges and 2 metadata columns
seqnames ranges strand score GC
<str> <IRanges> <ndarray[int8]> <range> <list>
[0] chr1 101 - 111 * | 0 0.9598854620837056
[1] chr2 102 - 122 - | 1 0.03155723261324739
[2] chr3 103 - 127 * | 2 0.6437812625719926
[3] chr2 104 - 133 + | 3 0.9111790719603508
[4] chr3 105 - 109 - | 4 0.9021352324834448
------
seqinfo(3 sequences): chr1 chr2 chr3
Inter-range methods¶
range: Returns a new
GenomicRanges
object containing range bounds for each distinct (seqname, strand) pair.reduce: returns a new
GenomicRanges
object containing reduced bounds for each distinct (seqname, strand) pair.gaps: Finds gaps in the
GenomicRanges
object for each distinct (seqname, strand) pair.disjoin: Finds disjoint intervals across all locations for each distinct (seqname, strand) pair.
gr = GenomicRanges(
seqnames=[
"chr1",
"chr2",
"chr3",
"chr2",
"chr3",
],
ranges=IRanges([x for x in range(101, 106)], [11, 21, 25, 30, 5]),
strand=["*", "-", "*", "+", "-"],
mcols=BiocFrame(
{
"score": range(0, 5),
"GC": [random() for _ in range(5)],
}
),
)
# range
range_gr = gr.range()
# reduce
reduced_gr = gr.reduce(min_gap_width=3, with_reverse_map=True)
# gaps
gapped_gr = gr.gaps(start=103) # OR
gapped_gr = gr.gaps(end={"chr1": 120, "chr2": 120, "chr3": 120})
# disjoin
disjoin_gr = gr.disjoin()
print("GenomicRanges with the disjoint ranges:")
print(disjoin_gr)
GenomicRanges with the disjoint ranges:
GenomicRanges with 5 ranges and 0 metadata columns
seqnames ranges strand
<str> <IRanges> <ndarray[int8]>
[0] chr1 101 - 111 *
[1] chr2 104 - 133 +
[2] chr2 102 - 122 -
[3] chr3 105 - 109 -
[4] chr3 103 - 127 *
------
seqinfo(3 sequences): chr1 chr2 chr3
/home/runner/work/GenomicRanges/GenomicRanges/.tox/docs/lib/python3.11/site-packages/genomicranges/SeqInfo.py:403: UserWarning: 'seqlengths' is deprecated, use 'get_seqlengths' instead
warn(
Set operations¶
union: Compute the
union
of intervals across objects.intersect: Compute the
intersection
or finds overlapping intervals.setdiff: Compute
set difference
.
#| code-fold: true
#| code-summary: "Show the code"
g_src = GenomicRanges(
seqnames = ["chr1", "chr2", "chr1", "chr3", "chr2"],
ranges = IRanges(start =[101, 102, 103, 104, 109], width=[112, 103, 128, 134, 111]),
strand = ["*", "-", "*", "+", "-"]
)
g_tgt = GenomicRanges(
seqnames = ["chr1","chr2","chr2","chr2","chr1","chr1","chr3","chr3","chr3","chr3"],
ranges = IRanges(start =range(101, 111), width=range(121, 131)),
strand = ["*", "-", "-", "*", "*", "+", "+", "+", "-", "-"]
)
# intersection
int_gr = g_src.intersect(g_tgt)
# set diff
diff_gr = g_src.setdiff(g_tgt)
# union
union_gr = g_src.union(g_tgt)
print("GenomicRanges after the union operation:")
print(union_gr)
GenomicRanges after the union operation:
GenomicRanges with 6 ranges and 0 metadata columns
seqnames ranges strand
<str> <IRanges> <ndarray[int8]>
[0] chr1 106 - 231 +
[1] chr1 101 - 230 *
[2] chr2 102 - 225 -
[3] chr2 104 - 227 *
[4] chr3 104 - 237 +
[5] chr3 109 - 239 -
------
seqinfo(3 sequences): chr1 chr2 chr3
Compute over bins¶
Summary stats for column¶
Use Pandas
to compute summary statistics for a column:
pd.Series(gr.mcols.get_column("score")).describe()
count 5.000000
mean 2.000000
std 1.581139
min 0.000000
25% 1.000000
50% 2.000000
75% 3.000000
max 4.000000
dtype: float64
With a bit more magic, render a histogram using matplotlib:
import numpy as np
import matplotlib.pyplot as plt
_ = plt.hist(gr.mcols.get_column("score"), bins="auto")
plt.title("'score' histogram with 'auto' bins")
plt.show()
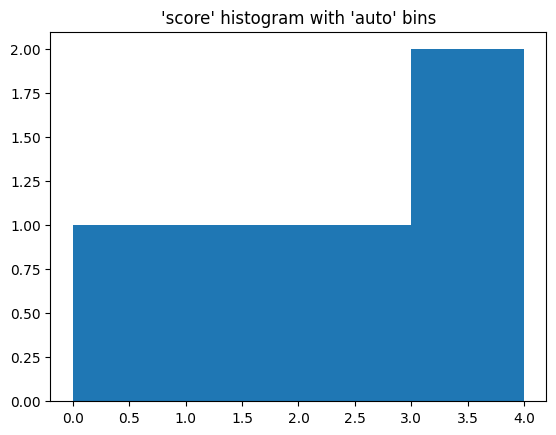
Not the prettiest plot but it works.
Binned average¶
Compute binned average for a set of query bins:
from iranges import IRanges
bins_gr = GenomicRanges(seqnames=["chr1"], ranges=IRanges([101], [109]))
subject = GenomicRanges(
seqnames= ["chr1","chr2","chr2","chr2","chr1","chr1","chr3","chr3","chr3","chr3"],
ranges=IRanges(range(101, 111), range(121, 131)),
strand= ["*", "-", "-", "*", "*", "+", "+", "+", "-", "-"],
mcols=BiocFrame({
"score": range(0, 10),
})
)
# Compute binned average
binned_avg_gr = subject.binned_average(bins=bins_gr, scorename="score", outname="binned_score")
print(binned_avg_gr)
GenomicRanges with 1 range and 1 metadata column
seqnames ranges strand binned_score
<str> <IRanges> <ndarray[int8]> <list>
[0] chr1 101 - 209 * | 3
------
seqinfo(1 sequences): chr1
Tip
Now you might wonder how can I generate these bins?
Generate tiles or bins¶
tile: Splits each genomic region by n (number of regions) or by width (maximum width of each tile).
sliding_windows: Generates sliding windows within each range, by width and step.
gr = GenomicRanges(
seqnames=[
"chr1",
"chr2",
"chr3",
"chr2",
"chr3",
],
ranges=IRanges([x for x in range(101, 106)], [11, 21, 25, 30, 5]),
strand=["*", "-", "*", "+", "-"],
mcols=BiocFrame(
{
"score": range(0, 5),
"GC": [random() for _ in range(5)],
}
),
)
# tiles
tiles = gr.tile(n=2)
# slidingwindows
tiles = gr.sliding_windows(width=10)
print(tiles)
[GenomicRanges(number_of_ranges=2, seqnames=[np.uint8(0), np.uint8(0)], ranges=IRanges(start=array([101, 102], dtype=int32), width=array([10, 10], dtype=int32)), strand=[np.int8(0), np.int8(0)], mcols=BiocFrame(data={}, number_of_rows=2, column_names=[]), seqinfoSeqInfo(number_of_seqnames=3, seqnames=['chr1', 'chr2', 'chr3'], seqlengths=[None, None, None], is_circular=[None, None, None], genome=[None, None, None])), GenomicRanges(number_of_ranges=12, seqnames=[np.uint8(1), np.uint8(1), np.uint8(1), ..., np.uint8(1), np.uint8(1), np.uint8(1)], ranges=IRanges(start=array([102, 103, 104, 105, 106, 107, 108, 109, 110, 111, 112, 113],
dtype=int32), width=array([10, 10, 10, 10, 10, 10, 10, 10, 10, 10, 10, 10], dtype=int32)), strand=[np.int8(-1), np.int8(-1), np.int8(-1), ..., np.int8(-1), np.int8(-1), np.int8(-1)], mcols=BiocFrame(data={}, number_of_rows=12, column_names=[]), seqinfoSeqInfo(number_of_seqnames=3, seqnames=['chr1', 'chr2', 'chr3'], seqlengths=[None, None, None], is_circular=[None, None, None], genome=[None, None, None])), GenomicRanges(number_of_ranges=16, seqnames=[np.uint8(2), np.uint8(2), np.uint8(2), ..., np.uint8(2), np.uint8(2), np.uint8(2)], ranges=IRanges(start=array([103, 104, 105, 106, 107, 108, 109, 110, 111, 112, 113, 114, 115,
116, 117, 118], dtype=int32), width=array([10, 10, 10, 10, 10, 10, 10, 10, 10, 10, 10, 10, 10, 10, 10, 10],
dtype=int32)), strand=[np.int8(0), np.int8(0), np.int8(0), ..., np.int8(0), np.int8(0), np.int8(0)], mcols=BiocFrame(data={}, number_of_rows=16, column_names=[]), seqinfoSeqInfo(number_of_seqnames=3, seqnames=['chr1', 'chr2', 'chr3'], seqlengths=[None, None, None], is_circular=[None, None, None], genome=[None, None, None])), GenomicRanges(number_of_ranges=21, seqnames=[np.uint8(1), np.uint8(1), np.uint8(1), ..., np.uint8(1), np.uint8(1), np.uint8(1)], ranges=IRanges(start=array([104, 105, 106, 107, 108, 109, 110, 111, 112, 113, 114, 115, 116,
117, 118, 119, 120, 121, 122, 123, 124], dtype=int32), width=array([10, 10, 10, 10, 10, 10, 10, 10, 10, 10, 10, 10, 10, 10, 10, 10, 10,
10, 10, 10, 10], dtype=int32)), strand=[np.int8(1), np.int8(1), np.int8(1), ..., np.int8(1), np.int8(1), np.int8(1)], mcols=BiocFrame(data={}, number_of_rows=21, column_names=[]), seqinfoSeqInfo(number_of_seqnames=3, seqnames=['chr1', 'chr2', 'chr3'], seqlengths=[None, None, None], is_circular=[None, None, None], genome=[None, None, None])), GenomicRanges(number_of_ranges=0, seqnames=[], ranges=IRanges(start=array([], dtype=int32), width=array([], dtype=int32)), strand=[], mcols=BiocFrame(data={}, number_of_rows=0, column_names=[]), seqinfoSeqInfo(number_of_seqnames=3, seqnames=['chr1', 'chr2', 'chr3'], seqlengths=[None, None, None], is_circular=[None, None, None], genome=[None, None, None]))]
Generate tiles from genome¶
tile_genome
returns a set of genomic regions that form a partitioning of the specified genome.
seqlengths = {"chr1": 100, "chr2": 75, "chr3": 200}
tiles = GenomicRanges.tile_genome(seqlengths=seqlengths, n=10)
print(tiles)
---------------------------------------------------------------------------
TypeError Traceback (most recent call last)
Cell In[19], line 3
1 seqlengths = {"chr1": 100, "chr2": 75, "chr3": 200}
----> 3 tiles = GenomicRanges.tile_genome(seqlengths=seqlengths, n=10)
4 print(tiles)
TypeError: GenomicRanges.tile_genome() got an unexpected keyword argument 'n'
Coverage¶
Computes number of ranges that overlap for each position.
import rich
res_vector = gr.coverage()
rich.print(res_vector)
Lets see what the coverage looks like, now with seaborn:
import seaborn as sns
vector = res_vector["chr1"]
sns.lineplot(data=pd.DataFrame({
"position": [i for i in range(len(vector))],
"coverage":vector
}), x ="position", y="coverage")
I guess that looks ok. :) but someone can help make this visualization better. (something that ports plotRanges
from R)
Overlap based methods¶
find_overlaps: Find overlaps between two
GenomicRanges
objects.count_overlaps: Count overlaps between two
GenomicRanges
objects.subset_by_overlaps: Subset a
GenomicRanges
object if it overlaps with the ranges in the query.
subject = GenomicRanges(
seqnames= ["chr1","chr2","chr2","chr2","chr1","chr1","chr3","chr3","chr3","chr3"],
ranges=IRanges(range(101, 111), range(121, 131)),
strand= ["*", "-", "-", "*", "*", "+", "+", "+", "-", "-"],
mcols=BiocFrame({
"score": range(0, 10),
})
)
df_query = pd.DataFrame(
{"seqnames": ["chr2",], "starts": [4], "ends": [6], "strand": ["+"]}
)
query = GenomicRanges.from_pandas(df_query)
# find Overlaps
res = subject.find_overlaps(query, query_type="within")
# count Overlaps
res = subject.count_overlaps(query)
# subset by Overlaps
res = subject.subset_by_overlaps(query)
print(res)
Search operations¶
nearest: Performs nearest neighbor search along any direction (both upstream and downstream).
follow: Performs nearest neighbor search only along downstream.
precede: Performs nearest neighbor search only along upstream.
find_regions = GenomicRanges(
seqnames= ["chr1", "chr2", "chr3"],
ranges=IRanges([200, 105, 1190],[203, 106, 1200]),
)
query_hits = gr.nearest(find_regions)
query_hits = gr.precede(find_regions)
query_hits = gr.follow(find_regions)
print(query_hits)
Note
Similar to IRanges
operations, these methods typically return a list of indices from subject
for each interval in query
.
Comparison, rank and order operations¶
match: Element-wise comparison to find exact match intervals.
order: Get the order of indices for sorting.
sort: Sort the
GenomicRanges
object.rank: For each interval identifies its position is a sorted order.
# match
query_hits = gr.match(gr[2:5])
print("matches: ", query_hits)
# order
order = gr.order()
print("order:", order)
# sort
sorted_gr = gr.sort()
print("sorted:", sorted_gr)
# rank
rank = gr.rank()
print("rank:", rank)
Combine GenomicRanges
objects by rows¶
Use the combine
generic from biocutils to concatenate multiple GenomicRanges
objects.
from biocutils.combine import combine
a = GenomicRanges(
seqnames=["chr1", "chr2", "chr1", "chr3"],
ranges=IRanges([1, 3, 2, 4], [10, 30, 50, 60]),
strand=["-", "+", "*", "+"],
mcols=BiocFrame({"score": [1, 2, 3, 4]}),
)
b = GenomicRanges(
seqnames=["chr2", "chr4", "chr5"],
ranges=IRanges([3, 6, 4], [30, 50, 60]),
strand=["-", "+", "*"],
mcols=BiocFrame({"score": [2, 3, 4]}),
)
combined = combine(a,b)
print(combined)
Misc operations¶
invert_strand: flip the strand for each interval
sample: randomly choose k intervals
# invert strand
inv_gr = gr.invert_strand()
# sample
samp_gr = gr.sample(k=4)
GenomicRangesList
class¶
Just as it sounds, a GenomicRangesList
is a named-list like object.
If you are wondering why you need this class, a GenomicRanges
object enables the
specification of multiple genomic elements, usually where genes start and end.
Genes, in turn, consist of various subregions, such as exons.
The GenomicRangesList
allows us to represent this nested structure.
As of now, this class has limited functionality, serving as a read-only class with basic accessors.
from genomicranges import GenomicRangesList
a = GenomicRanges(
seqnames=["chr1", "chr2", "chr1", "chr3"],
ranges=IRanges([1, 3, 2, 4], [10, 30, 50, 60]),
strand=["-", "+", "*", "+"],
mcols=BiocFrame({"score": [1, 2, 3, 4]}),
)
b = GenomicRanges(
seqnames=["chr2", "chr4", "chr5"],
ranges=IRanges([3, 6, 4], [30, 50, 60]),
strand=["-", "+", "*"],
mcols=BiocFrame({"score": [2, 3, 4]}),
)
grl = GenomicRangesList(ranges=[a,b], names=["gene1", "gene2"])
print(grl)
Properties¶
grl.start
grl.width
Combine GenomicRangeslist
object¶
Similar to the combine function from GenomicRanges
,
grla = GenomicRangesList(ranges=[a], names=["a"])
grlb = GenomicRangesList(ranges=[b, a], names=["b", "c"])
# or use the combine generic
from biocutils.combine import combine
cgrl = combine(grla, grlb)
The functionality in GenomicRangesLlist
is limited to read-only and a few methods. Updates are expected to be made as more features become available.
Empty ranges¶
Both of these classes can also contain no range information, and they tend to be useful when incorporates into larger data structures but do not contain any data themselves.
To create an empty GenomicRanges
object:
empty_gr = GenomicRanges.empty()
print(empty_gr)
Similarly, an empty GenomicRangesList
can be created:
empty_grl = GenomicRangesList.empty(n=100)
print(empty_grl)
Futher reading¶
Check out the reference documentation for more details.
Visit Bioconductor’s GenomicRanges package.